Executive Summary
The AI industry has a low threat of new entrants due to its technical and capital capabilities requirement to develop AI. This explains why only the big tech companies are leading the whole market. The bargaining power of suppliers is high due to the GPU share being mainly dominated by Nvidia and the access to proprietary data to train AI can not be easily found. Moreover, many big tech create their own GPU cloud service giving them have more competitive advantage than others. AI development is an industry with high competitive rivalry. The competition is not only for large customers but also in the market of inputs, model development and labor. Enterprises have to be in fierce competition to attract and retain employees, especially employees with experience and skills.
1. Current AI Trend
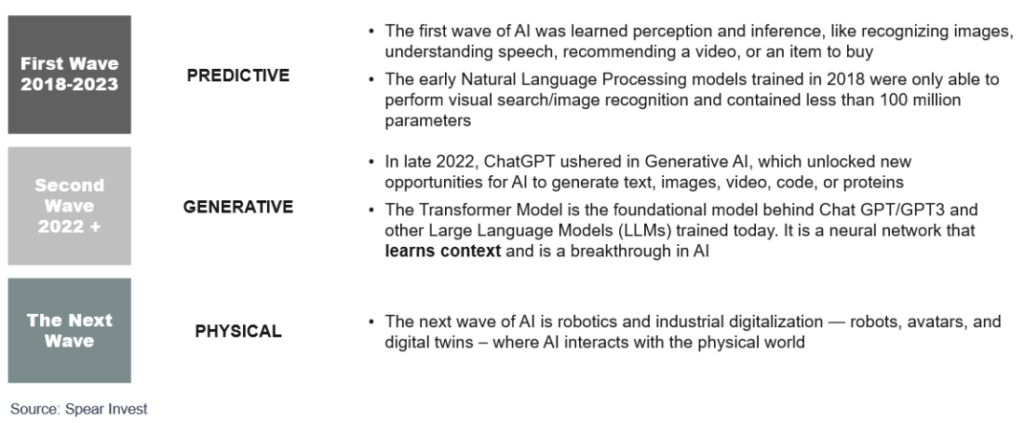
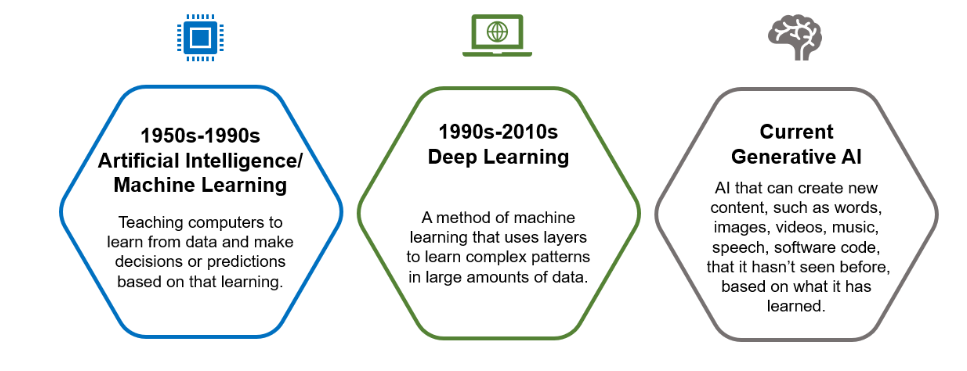
Generative AI, as characterized by Spear Invest and supported by Oppenheimer, encompasses algorithms that produce original content, including text, images, videos, music, speech, and software code, drawing from learned data patterns. This technology challenges the traditional view that creativity is uniquely human by utilizing complex data interpretations to create innovative outputs. Currently, generative AI is at the cutting edge of AI development, exemplified by platforms like ChatGPT, which continuously improve through interactions with over one billion unique monthly users globally. This rapid progression highlights the technology’s significant potential and sets the stage for future advancements where AI will increasingly interact with the physical world, as projected by Spear Invest.
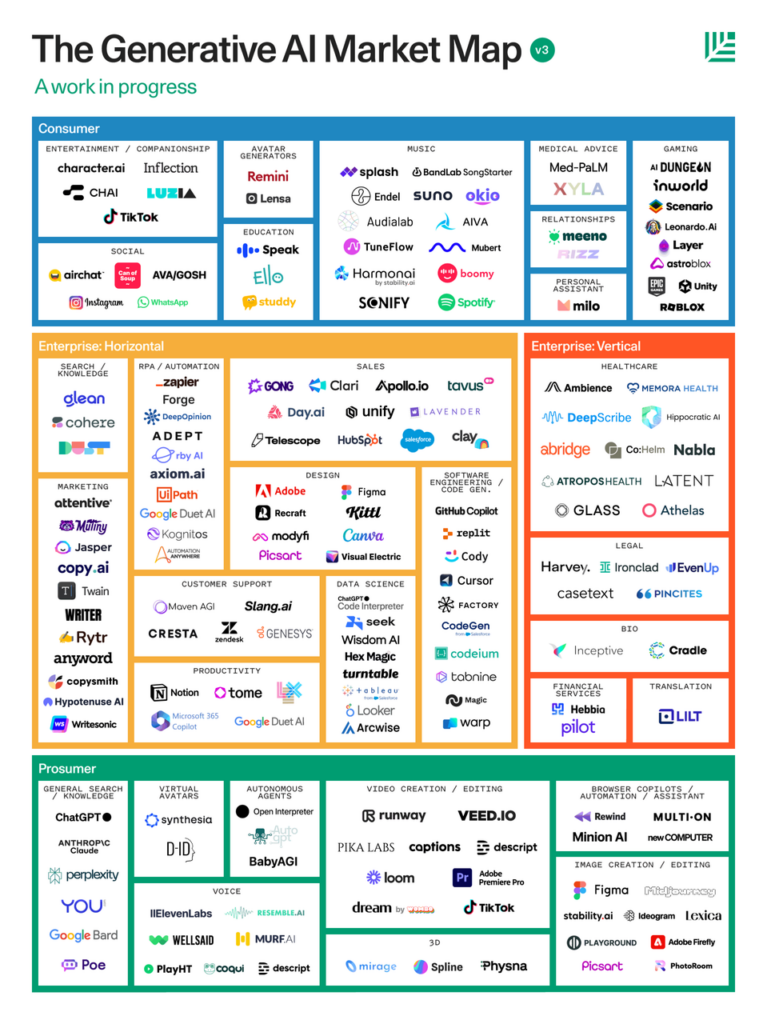
Despite a broader downturn in AI private investment last year, funding for generative AI—a subset of artificial intelligence that focuses on content creation—experienced significant growth. In 2023, the sector attracted $25.2 billion in investments, representing nearly a ninefold increase from 2022 and a thirtyfold rise from 2019. This surge underscores robust investor confidence and expanding market opportunities in the generative AI field.
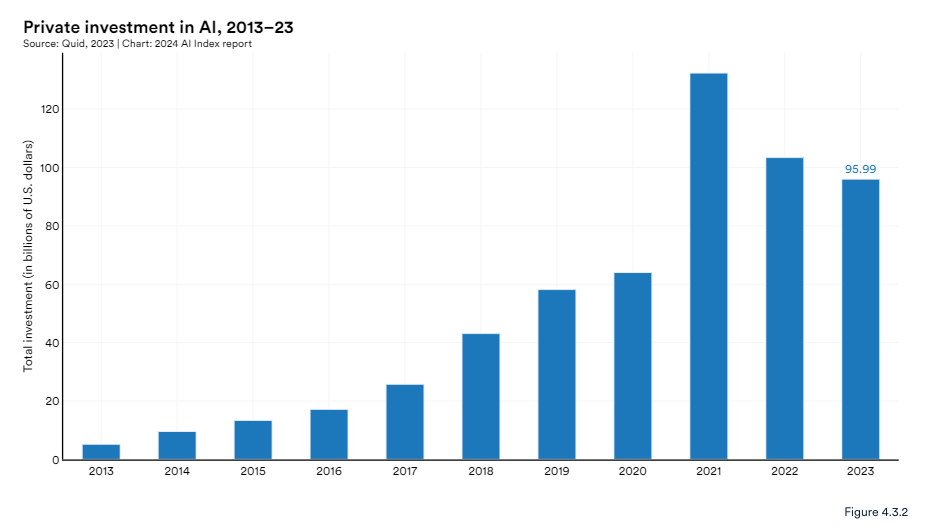
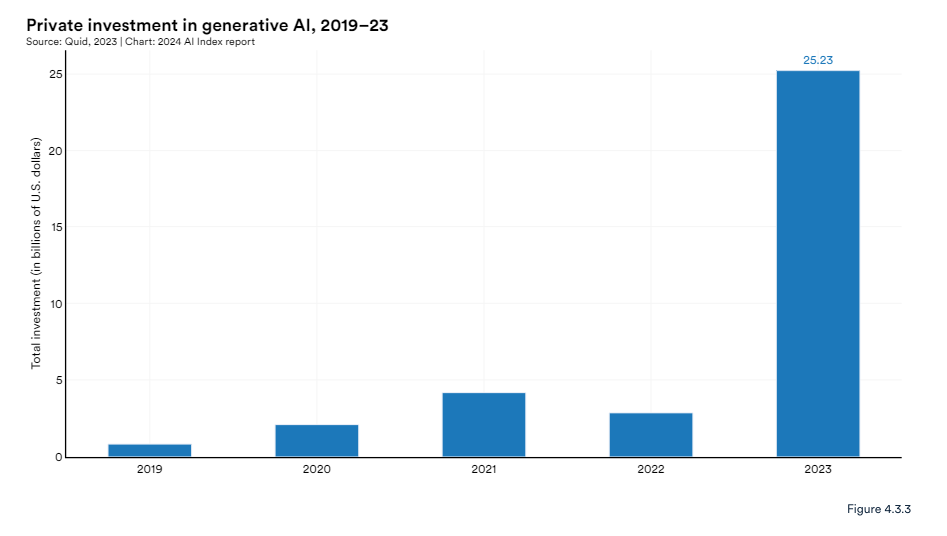
In 2023, generative AI accounted for over 25% of all AI-related private investments. The average investment size in this sector was calculated by dividing the total annual private investment in AI by the number of investment events. Between 2022 and 2023, the average investment size in generative AI slightly increased from $31.3 million to $32.4 million.
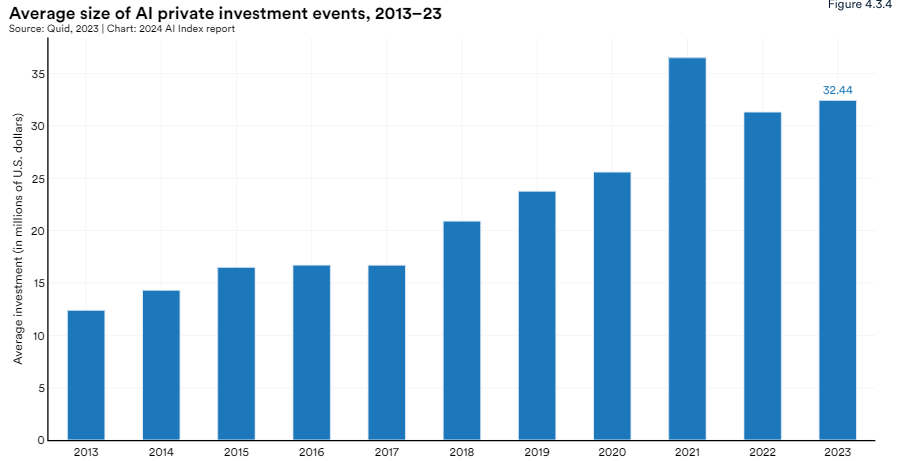
2. Supply and Demand in AI
2.1. Supply
GPU Supply
H100 and A100 chips, crucial for AI training, are primarily acquired by leading technology firms, with the United States maintaining the largest share of the global AI chip market. In late August 2022, these chips, notably NVIDIA’s A100 and H100—some of the most sophisticated for AI applications—were added to the US Commerce Department’s export control list. This measure restricts Chinese companies from accessing these advanced technologies.
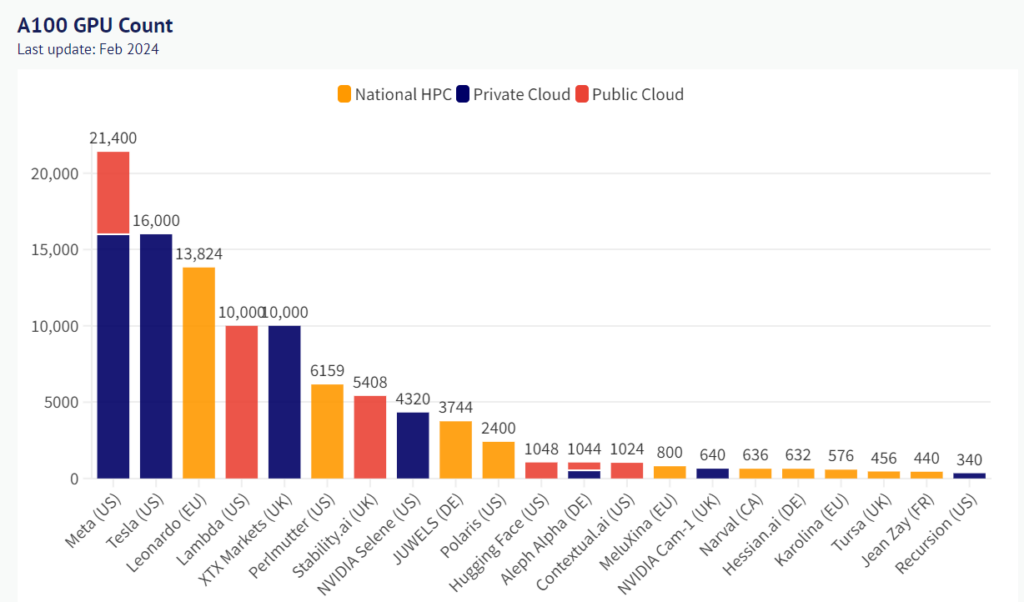
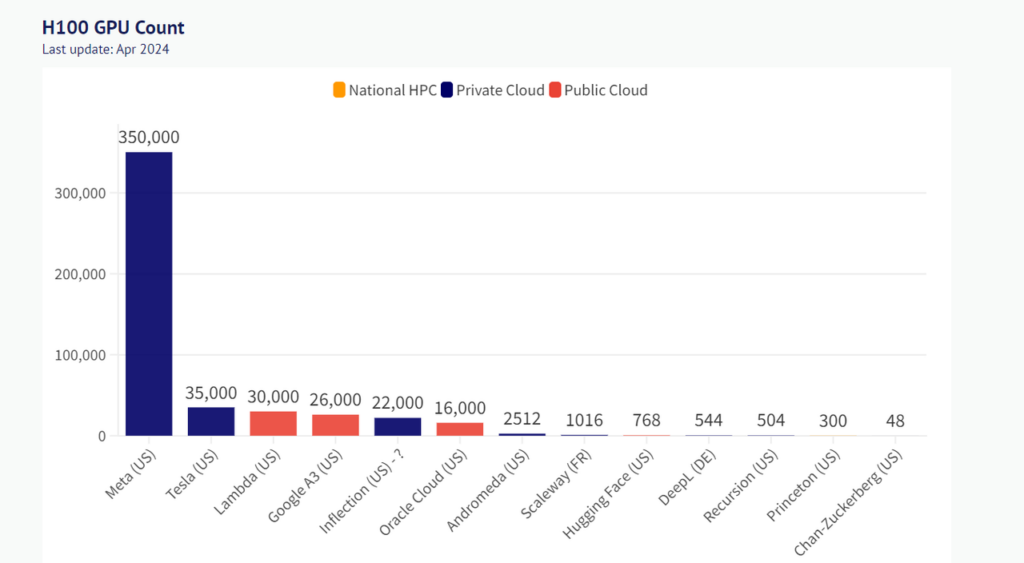
More demand for bandwidth
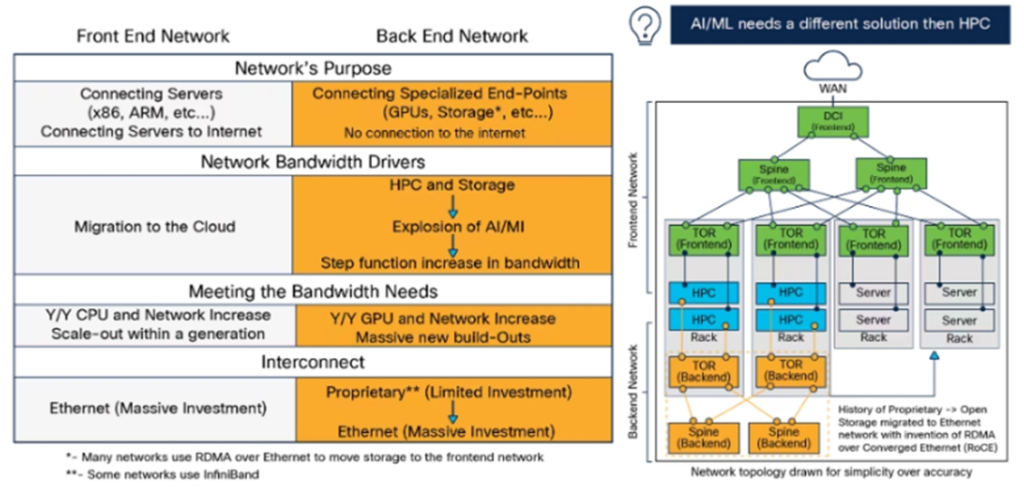
AI models require extensive bandwidth to manage large-scale data transfers and computational processes necessary for accessing training materials. This need for a constant and robust internet connection must support substantial data downloads from various global locations without interruption. The resulting high demand can lead to network congestion, packet loss, latency, and disruptions in model training or operational inference. According to industry analyst Lightcounting, sales of optical transceivers specifically for AI clusters are expected to reach $17.6 billion over the next five years. This is noteworthy as it comprises a significant portion of the $28.5 billion forecasted for all Ethernet transceiver applications combined during the same period.
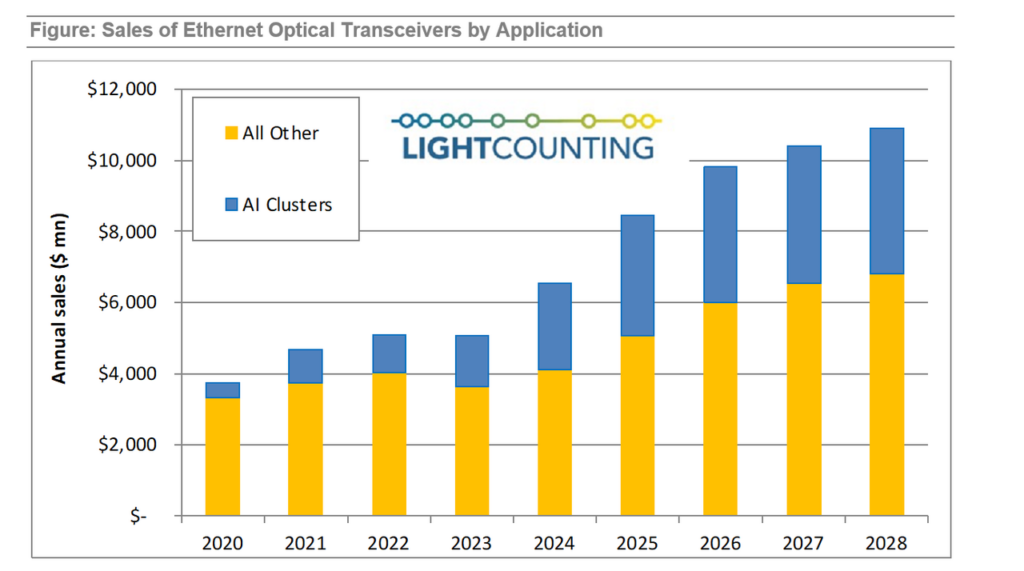
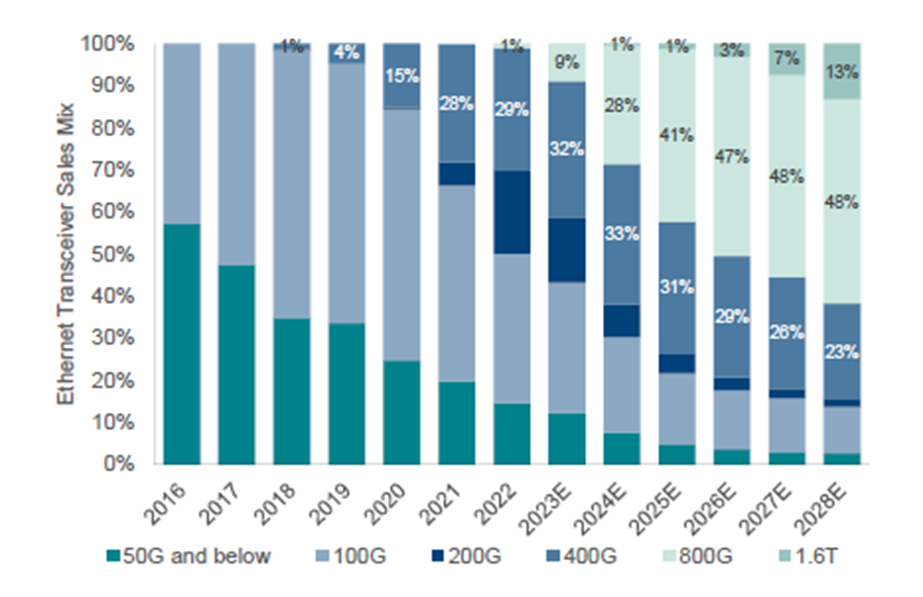
The shortage of data to train AI
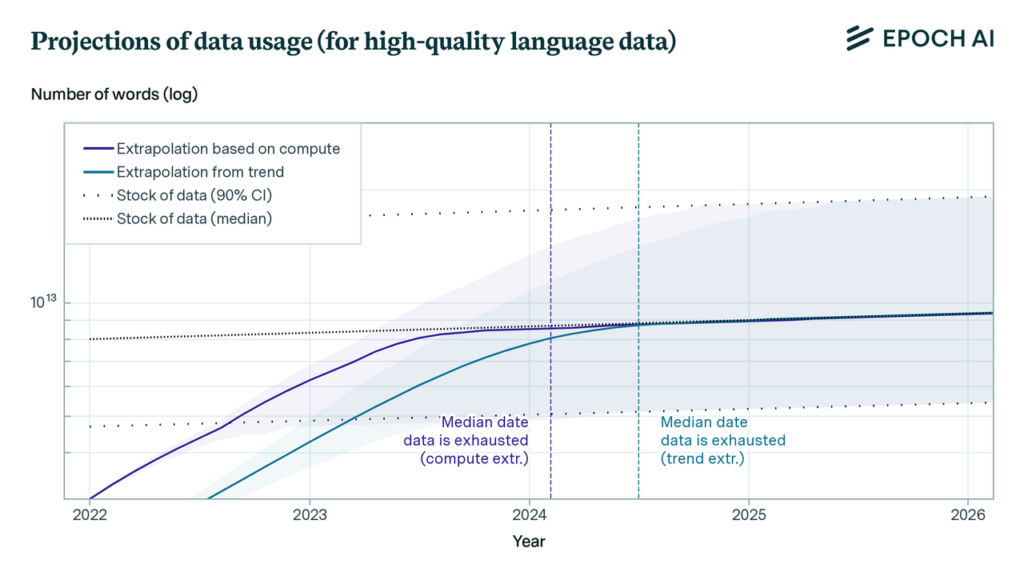
Data dependency in artificial intelligence signifies the reliance of AI models on substantial volumes of data for their training and ongoing enhancement. As AI technology progresses, the sufficiency of accessible data is becoming a critical issue. EpochAI predicts that by 2030 to 2050, low-quality language data will be depleted, high-quality language data will be exhausted by 2026, and vision-related data will be unavailable between 2030 and 2060. This looming scarcity of essential data resources could impede advancements in machine learning, presenting significant obstacles to future innovations in the field.
AI salary in the US is significantly higher
Salaries vary by role and geographic location. Globally, a cloud infrastructure engineer earns an average salary of $105,000; in the United States, this figure increases to $185,000. Senior executives and engineering managers typically receive the highest compensation, both in the U.S. and internationally. Overall, the United States consistently offers higher salaries across various roles compared to other nations.
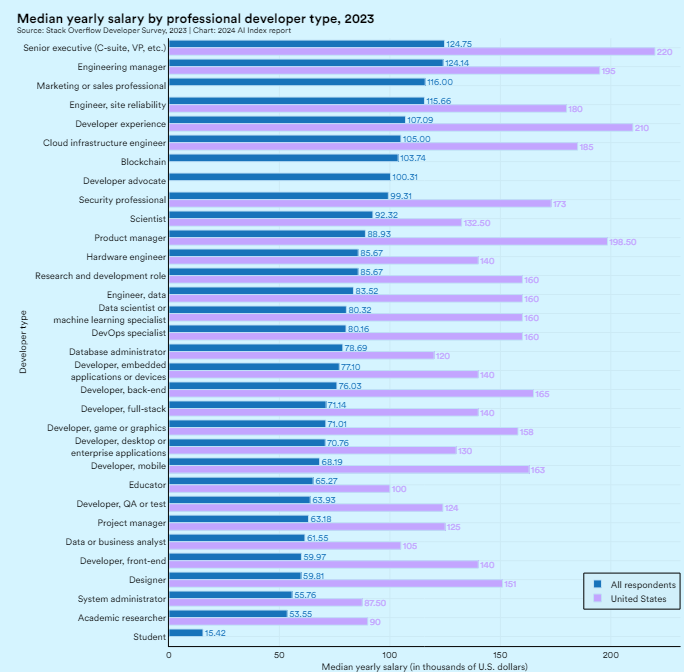
The increase in training cost
There is a discernible correlation between the size of large language and multimodal models and their associated costs.
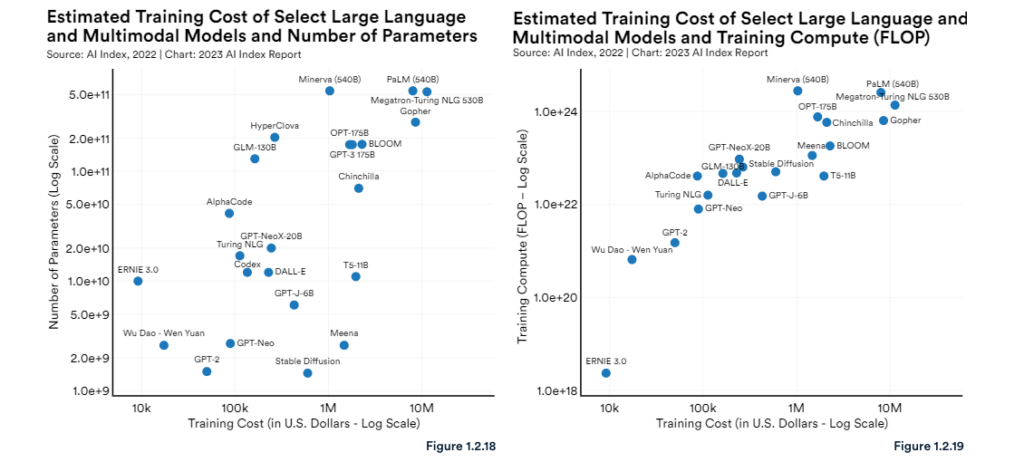
The Stanford AI Index report highlights the substantial and increasing costs of training AI models, often reaching millions of dollars. This upward trend is detailed through an analysis of training durations, hardware types, quantities, and utilization rates, based on data from publications, press releases, and technical reports. AI computation costs are divided into two primary categories: hardware costs, representing the proportion of initial hardware expenditure used during training, and energy costs, covering the electricity required to operate the hardware throughout the training period. The report demonstrates a significant rise in these costs over time, emphasizing the escalating financial demands of advancing AI technologies.
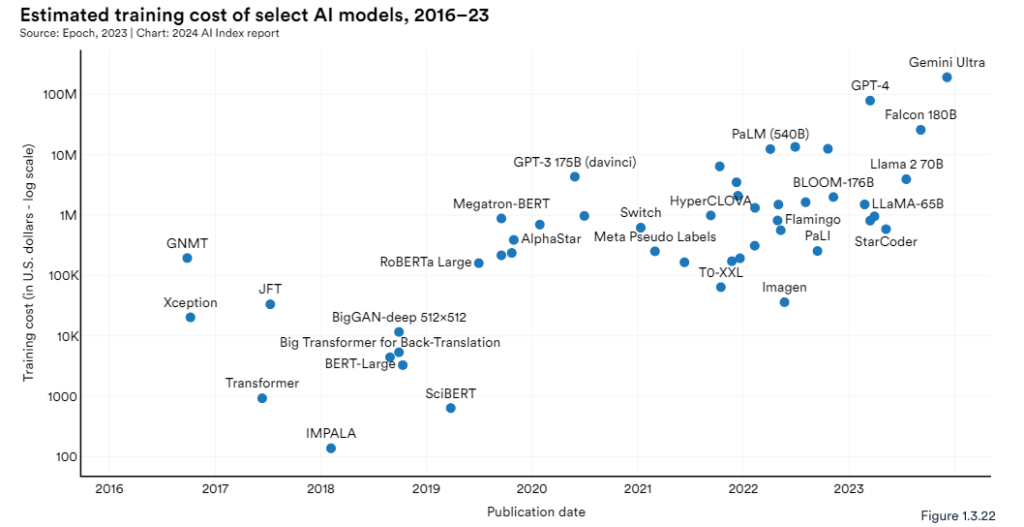
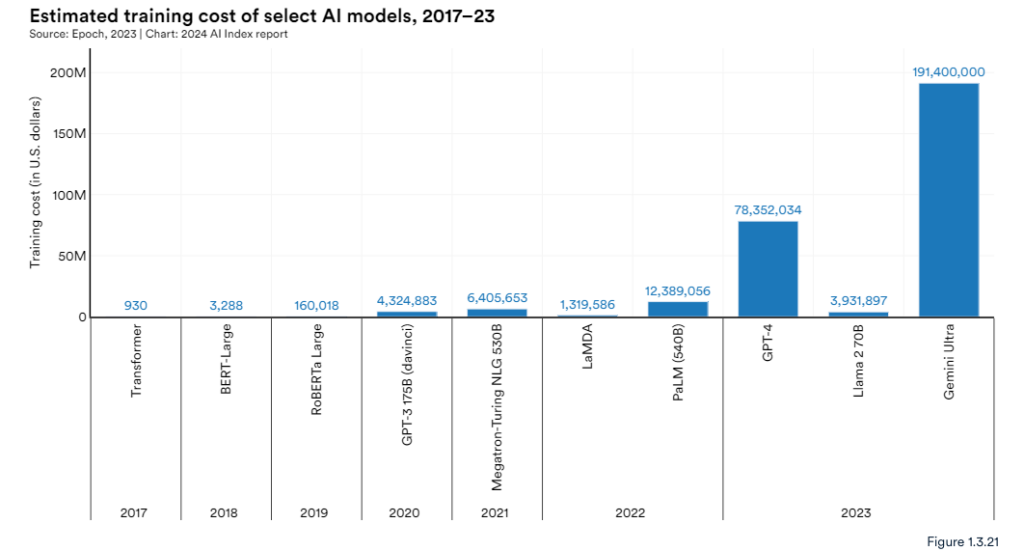
2.2. Demand
AI adoption
In 2023, the tech, media, and telecom sectors led AI adoption, with 44% of companies integrating AI in product and service development. Service operations and marketing and sales saw significant AI usage, each at 36%. AI was frequently deployed across multiple functions, with key use cases including contact center automation (26%), personalization (23%), customer acquisition (22%), and AI-enhanced product features (22%).
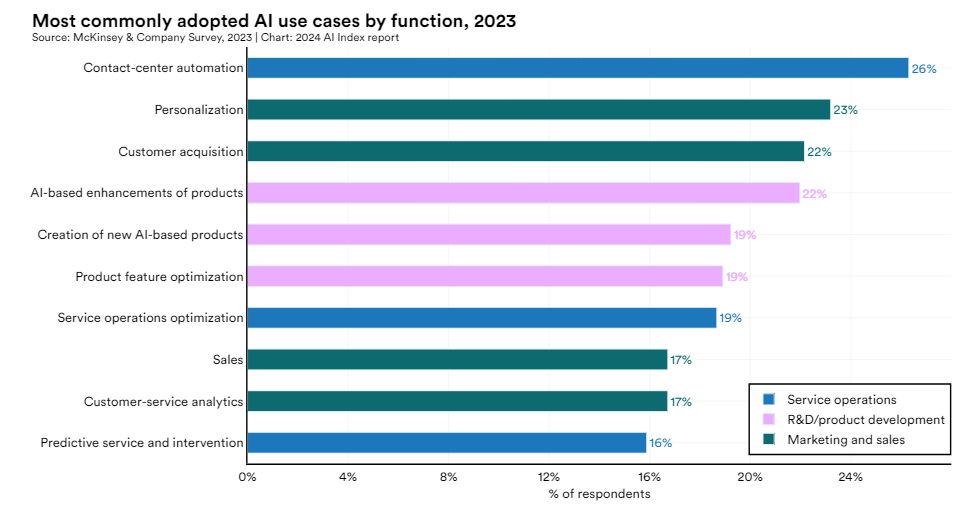
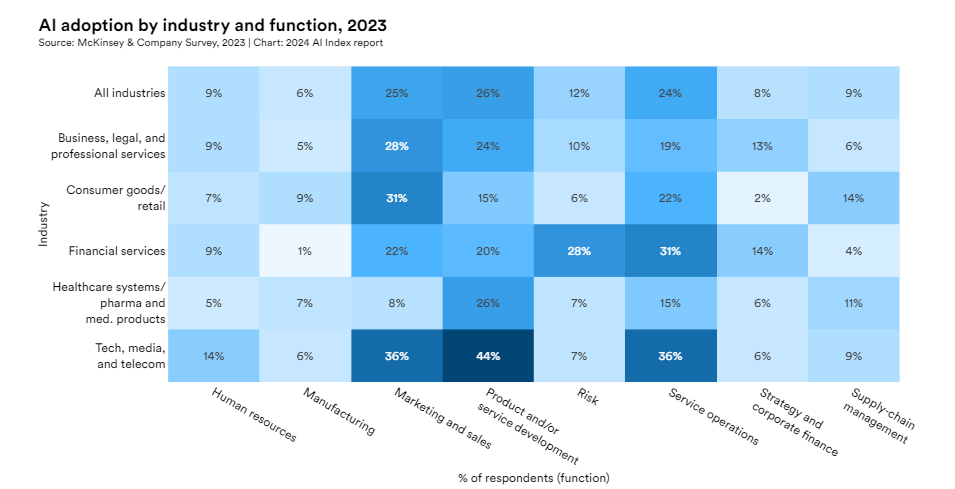
3. AI Competitive Environment
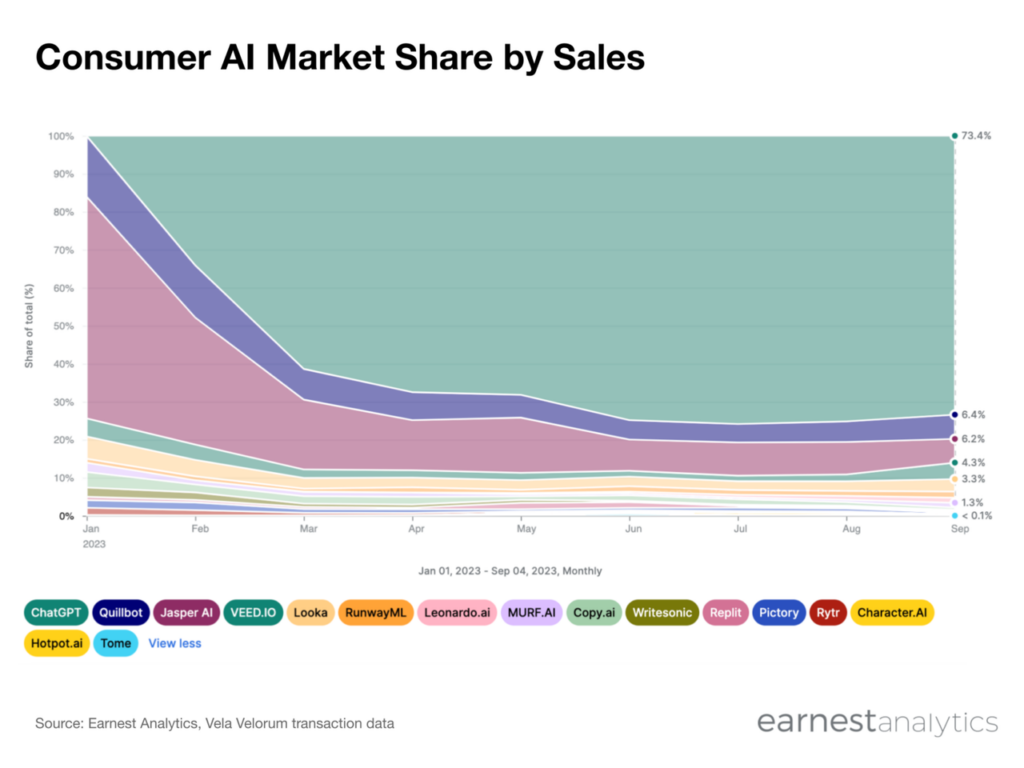
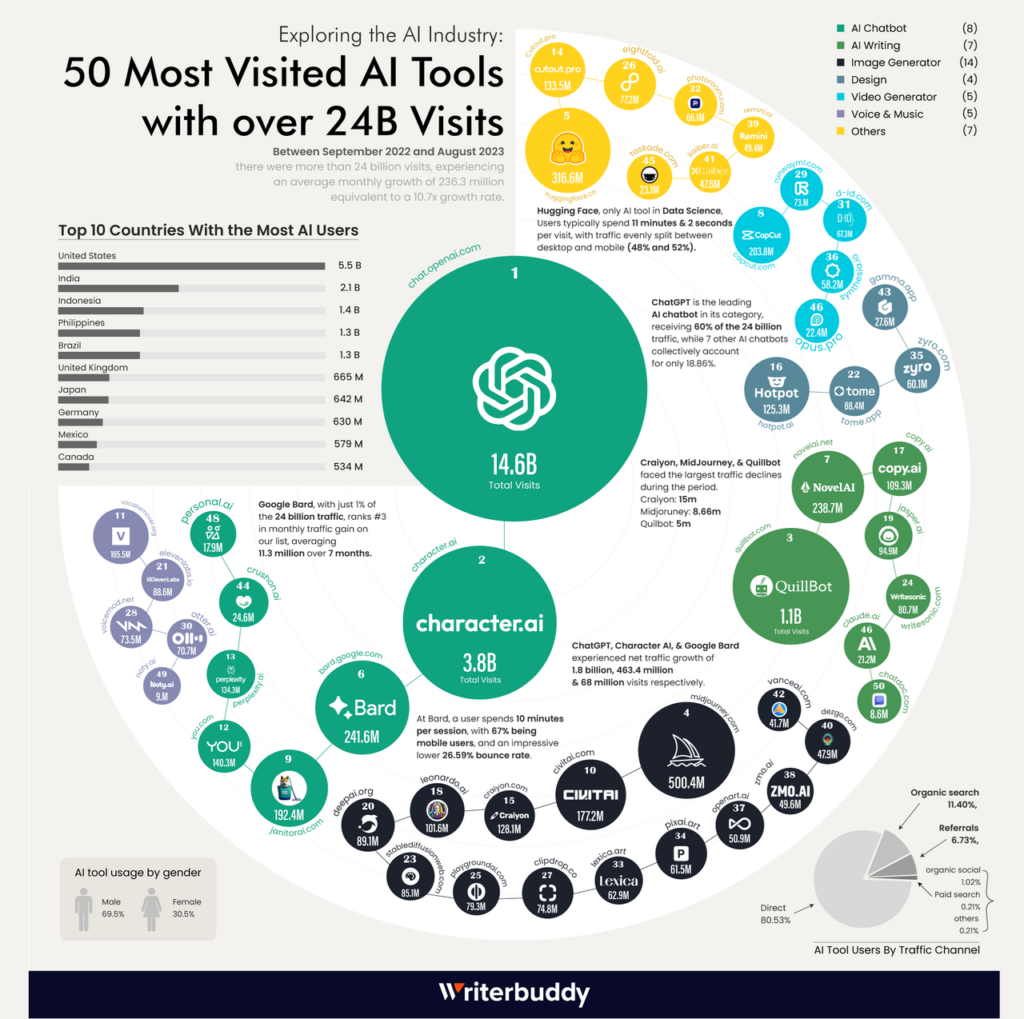
In 2023, consumer AI tools saw remarkable growth, with payments to 16 major platforms rising by 570% year-over-year in August. ChatGPT led the market, capturing over 73% of consumer AI sales after launching a free tool earlier in the year, surpassing the previously dominant Jasper AI. ChatGPT’s success is attributed to its competitive average ticket price of $20, which facilitated the conversion of freemium users to paying customers. In contrast, Jasper AI maintained a high average ticket price of $108 in August. RunwayML, though holding less than 1.5% of the market, experienced the fastest growth with a 1700% year-over-year increase.
Foundation models are crucial to artificial intelligence, acting as the core frameworks for diverse AI applications. Google leads this field, having released 40 foundation models since 2019, outpacing OpenAI’s 20 releases. Among non-Western entities, Tsinghua University stands out with seven releases, while Stanford University leads in the U.S. academic sector with five releases.
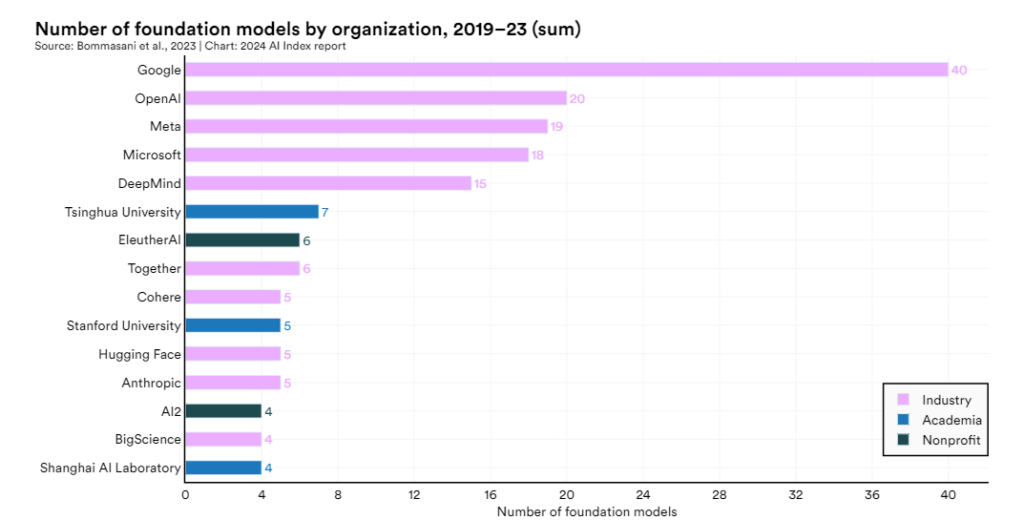
The Holistic Evaluation of Language Models (HELM) framework assesses large language models (LLMs) across various scenarios, including reading comprehension, language understanding, and mathematical reasoning. HELM evaluates models from leading companies like Anthropic, Google, Meta, and OpenAI using a “mean win rate” metric, which measures average performance across all tested scenarios. As of January 2024, OpenAI’s GPT-4 tops the HELM aggregate leaderboard with a mean win rate of 0.96. While GPT-4 leads overall, other models excel in specific tasks. Notably, Palmyra from AI startup Writer ranks third and fourth, followed by Google’s PaLM-2 in fifth place.
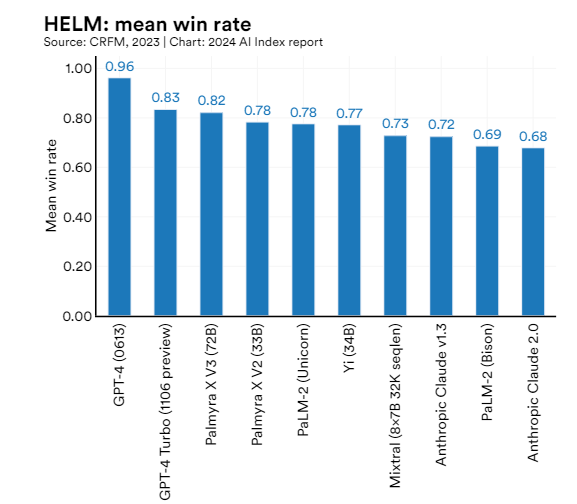
The SWE-bench is a stringent benchmark for assessing AI coding proficiency, requiring systems to handle updates across various functions, interact with multiple execution environments, and perform complex reasoning. Despite these challenges, state-of-the-art large language models (LLMs) have generally struggled. In 2023, Claude 2 achieved the highest performance, solving just 4.8% of the tasks, which is a 4.3 percentage point improvement over the previous year’s best model. Meta’s Llama 13B and 7B secured the second and third positions, respectively, while Anthropic’s model ranked fourth, resolving 1.96% of the challenges.
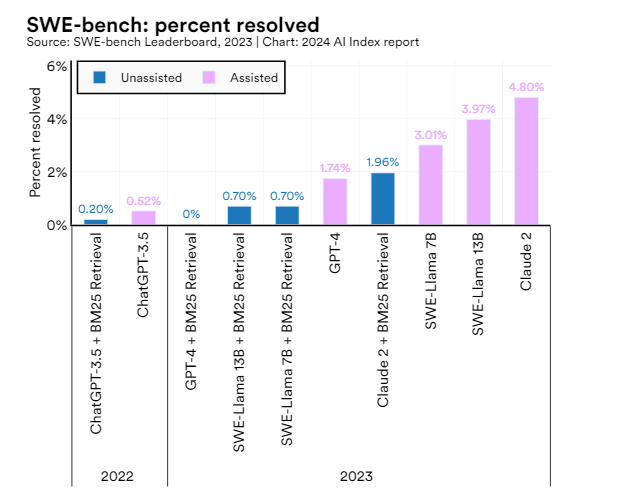
The Holistic Evaluation of Text-to-Image Models (HEIM) is a comprehensive benchmark designed to rigorously assess image generators across 12 key dimensions critical for practical application, including image-text alignment, image quality, and aesthetics. Utilizing human evaluators, HEIM addresses the limitations of automated metrics that often fail to effectively capture nuanced image attributes. Current HEIM findings indicate that no single model excels across all categories. OpenAI’s DALL-E 2 is the leader in image-to-text alignment, ensuring the best correspondence between generated images and input text. Conversely, the Stable Diffusion-based Dreamlike Photoreal model scores highest in image quality, aesthetics, and originality, producing visually appealing images that closely resemble real-life photography while maintaining uniqueness and adhering to copyright norms.
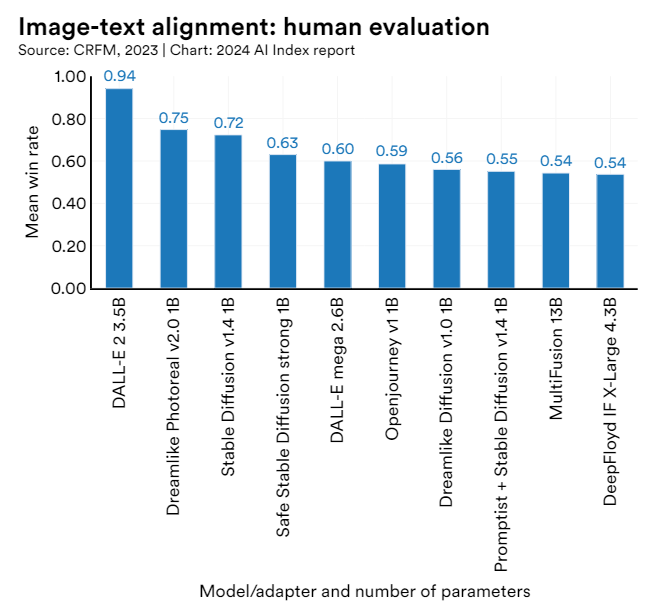
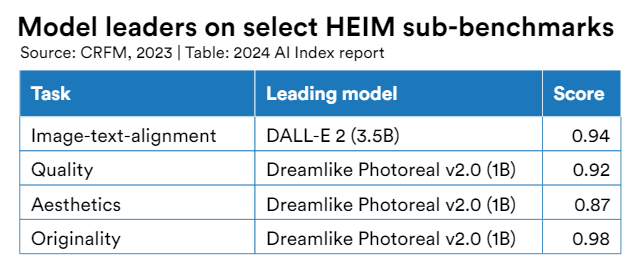
“Small language models” challenge larger rivals
Large language models (LLMs) are advanced generative AI systems known for their extensive computational requirements. However, they are increasingly facing competition from Small Language Models (SLMs), which require significantly less computational power. SLMs can operate efficiently, economically, and securely on personal devices, eliminating the need for cloud-based platforms. Apple and Microsoft have recently advanced the market for these efficient models. Apple has introduced eight OpenELMs—open-source Efficient Language Models designed to run directly on its devices, potentially integrating AI capabilities into iOS. Concurrently, Microsoft launched Phi-3 mini, a compact model with 3.8 billion parameters, which is only 0.2% the size of OpenAI’s GPT-4 but is touted to be ten times more cost-effective for comparable tasks.
According to Deutsche Bank Research, this shift is significant because large AI models, despite their robust capabilities, are costly and slow, often requiring fine-tuning to avoid generic outputs. In contrast, smaller AI models offer cost efficiency, enhanced speed, personalization, and increased security. Extended training periods using larger datasets can significantly improve the efficiency of models with fewer parameters, aligning performance more closely with user-specific needs. This approach optimizes AI applications by balancing sophistication with practical deployment demands.
The information provided in this article is for reference only and should not be taken as investment advice. All investment decisions should be based on thorough research and personal evaluation.
[…] AI Industry […]
[…] AI Industry […]
Comments are closed.